- September 22, 2023
Can AI be censored for causing exclusion, false predictions?
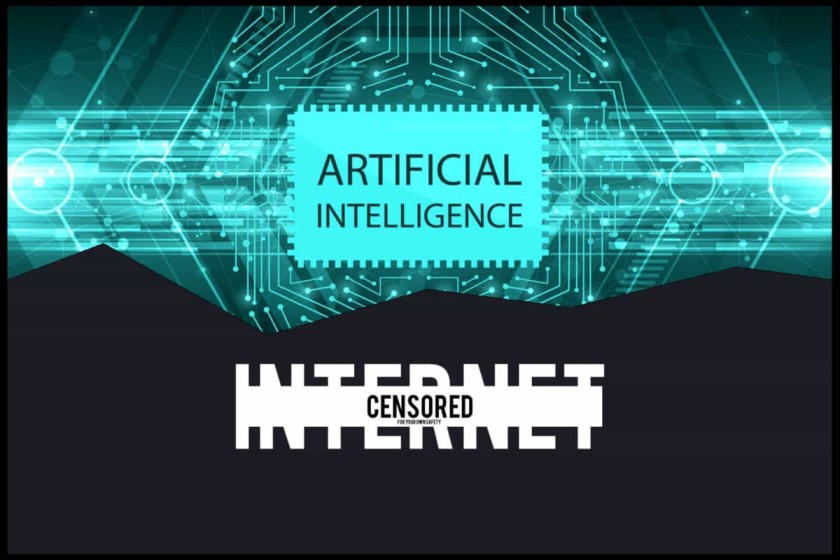
Explore India’s Artificial Intelligence challenges in exclusion and false predictions, with a multi-stakeholder approach to responsible AI governance.
One of the main concerns around Artificial Intelligence is the production of biased outputs and false predictions, which could lead to the exclusion of impact populations, traditionally excluded in real life. India is a diverse and complex country with historic dispositions like patriarchy, caste discrimination, etc.
However, when AI reflects society like a mirror, can we censure AI for causing exclusion and false predictions? Answering this question, in our recent study, we highlighted how AI technology is not harmful, but rather, it replicates biases due to the biases present in society and how the algorithms are designed.
How exclusion happens
Our study differentiates between harms (the actual negative consequences that may arise from AI systems) and impacts (evaluative constructs used to gauge the socio-material harms) emerging at different stages of the AI lifecycle. The objective of doing this is to develop a map of harms and impacts caused by different stakeholders at different stages of the AI lifecycle.
The study reveals that the potential danger of exclusion caused by AI is not just at the development stage but also at the deployment level, where harm could be caused by AI deployers who may abuse and misuse the technology.
At the development stage, predominantly cognitive biases, historical biases, representation biases, measurement biases, etc., subjected to humans, shape the developed AI technology, ultimately leading to exclusionary outcomes.
The human brain simply processes information by prioritising preferred outcomes due to cognitive biases. However, in the AI scenario, cognitive biases could bring out exclusionary implications.
For instance, hypothetically, if the individuals involved in ideating an AI solution are exposed to patriarchal socialisation, their biases may seep into the AI solution, leading to the exclusion of women as an outcome. Similarly, the exclusion could happen even if the dataset is appropriately measured and sampled because of historical bias, where data carries biases as it is.
Moreover, deployment bias could bring exclusion even if an AI technology is developed with all the precautions. This happens when the AI deployers employ the AI solutions for a different purpose than what it was created for based on the judgment of human decision-makers, also called the framing trap.
For instance, while some prediction technologies in legal enforcement are developed for recidivism, it was noted that such AI solutions are also used to determine the length of the sentence. Also, when the AI technologies produce exclusionary outputs, the confirmation bias of the AI deployers, i.e., confirming their existing belief, might blind them from noticing the error, causing exclusion.
Why AI makes false predictions
As discussed, in the Indian context, the presence of a historically biased disposition against certain groups could aggravate adverse implications of the AI systems, like false predictions. While false predictions are one half of the story creating impact, our study shows that the second half is when the AI deployers use those false predictions daily for determining eligibility, profiling, etc, causing entry barriers and discrimination.
As a human tendency, we borrow innovations and ideas from different scenarios and streams into our work field subject to those innovations’ success rate. However, in this process, we might overestimate the capacity of such innovation and not consider the incompatibility of the same within specific sectors.
For instance, while AI-based predictive technologies are extensively used in weather predictions and meteorology, it is not necessarily true that similar technology would work completely prejudice-free when used for predicting recidivism. Besides, at the deployment stage, AI users are subjected to automation biases where they believe the computational results of an AI model are accurate, which may lead to them blindly relying on the results of such an AI model.
Charting the way forward
To tackle the implications of AI, various policy developments have emerged worldwide to enhance AI risk management to make the technology responsible. However, many of these frameworks largely focus on a uni-stakeholder, i.e., AI developers.
However, our study highlights that at different points within the AI lifecycle stage, a particular stakeholder, including AI developers, AI deployers and impact population/end-users, contributes in different forms, ultimately adding to the causation of critical implications like exclusion and false predictions.
Therefore, as the use case of AI continues to evolve, we propose a “Principle-Based Multi-Stakeholder Approach” as an ecosystem-level intervention to make AI technologies responsible.
The principle-based multi-stakeholder approach discusses various principles across the AI lifecycle bucketed and mapped to respective stakeholders within the AI ecosystem. Through this, we underscore the importance of fostering responsible AI within India’s ecosystem, involving all stakeholders, including developers, deployers, and users.
The framework contributes to shaping an effective governance structure for AI, focusing on multi-stakeholder engagement to imbibe responsible principles throughout the AI lifecycle. This also aligns with the G-20 New Delhi Leaders’ Declaration.
Kamesh Shekar is Programme Manager, The Dialogue, and leads the data governance vertical. Jameela Sahiba is Senior Programme Manager, The Dialogue. Views are personal, and do not represent the stand of this publication.