- July 22, 2023
The future of AI: Will AI models go big or small?
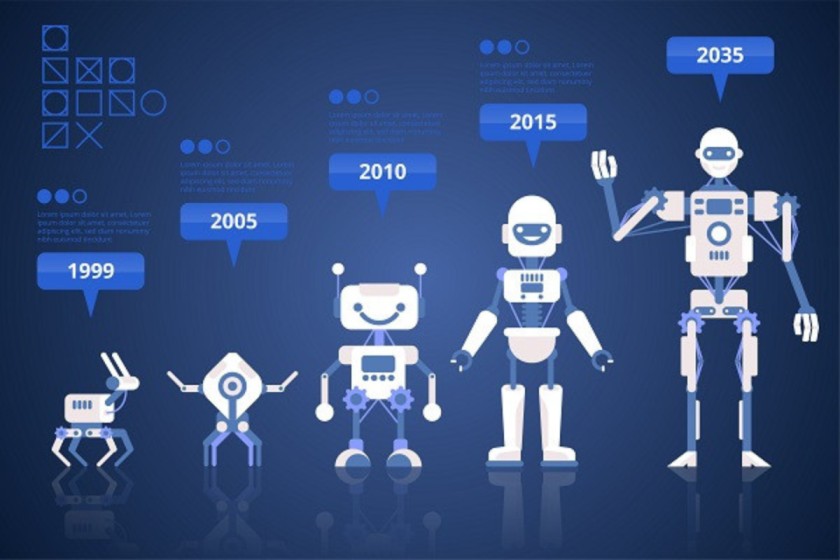
AI’s versatility poses cost challenges, but factors like fine-tuning, shrinking data needs, and free models are driving innovation.
Foundational AI models by Microsoft, Google, Meta, and the like have captivated everyone from businesses to casual observers. This is because their creativity and articulation abilities are wide enough to satisfy general curiosity, while also being customisable enough to satiate business demands.
However, developing this versatility hasn’t been cheap. Myriad steep costs including those for obtaining quality data, hardware, electricity, and talent have all stood as tall barriers in the training and deployment of large AI models.
Despite these steep costs posing a major hindrance for AI development, innovation within the AI space has continued unabated. This is because various factors have coalesced to allow a thriving small AI ecosystem to develop. This article explores some of these and, using that information, postulates how the future of this AI sub-sector could pan out.
Why AI’s catching on
The first thing to appreciate about AI adoption is that the pace will only increase here onwards. This is because of various tangible benefits that it offers, in terms of knowledge management and productivity enhancement.
By adopting language models into their processes, firms can aggregate their disparate data sources like online chats, meetings, operational transactions, policy documents etc., and gain insights into various domains.
This is equivalent to a mining firm recognising that the slag (a byproduct of mining) which they produce also has value, along with understanding the process by which they could extract this said value. Any organisation would want to jump aboard such opportunities. The only question that they would have is – can they do it, and what will it cost.
And in the case of AI, there are a few factors that are working to reduce the cost barrier of adoption.
Fine-tuning off-the-shelf models
The first trend driving cost reduction is the fine-tuning of AI models. While it is not the cheapest solution around, it is still more frugal than training an in-house language model. In this approach, an organisation uses an API to access an existing foundational model like GPT-4, and then tweaks it using their own data to optimise it for their domain-specific needs.
Examples of this approach range from the pricey solutions of Morgan Stanley, which incorporated one lakh of its research reports to train GPT-4, to Stanford’s Alpaca model which was trained for a mere $600 by fine-tuning Meta’s LLaMA model.
Considering the low-cost of Stanford’s model along with its competence (it can go toe-to-toe with GPT-3.5 in various tests, which cost several million dollars to train), we can see the power of this approach.
Edge AI
Another factor driving AI innovation and helping the growth of small models is Edge AI. Till now, if you wanted to train your own model and run it, you would have had to utilise the services of a centralised cloud computing facility.
Now, however, with greater AI computational power being researched and introduced by firms like Qualcomm (whose chips power most of the Android phones in the world), models that are up to ten billion parameters in size could soon become executable on the phone itself. This has been dubbed as Edge AI
This democratisation of unprecedented computational power, along with the proliferation of competent small AI models is the perfect recipe for mass AI adoption. Soon, we could have a situation where normal people are able to run models as potent as GPT-3.5 locally on their phone with no internet access. That could really be a game-changer.
Training data shrinks
Innovation and adoption have also been aided by shrinking data requirements for training AI models. This has been accomplished by a method called transfer learning, where a pre-trained model gets reused to solve new problems.
For example, using a readily available model that is pre-trained on millions of images (like the ImageNet database), a clinic could start to conduct kidney disease diagnosis by training it on only a handful of images of kidneys. Various medical papers have, in fact, highlighted how this can be accomplished, including some from India.
OpenAI’s Codex is another example that reveals the power of this technique. This model powers GitHub’s Copilot, which is a programming tool that writes code based on the prompts it gets. By January 2023, it had already racked up over a million subscribers, and it has been trained using GPT-3.
Codex has been easily able to surpass GPT-3’s coding ability while being trained on a dataset that is hundreds of times smaller, due to transfer learning. While GPT-3 took 45 terabytes of data to train, Codex took only 159 GB. Thus, we can see that where large pre-trained models can be leveraged, data requirements shrink dramatically.
The free large models
A fourth factor that has driven growth in the AI space is the wide proliferation of free, large AI models. Whether it is Microsoft’s Orca, which has been taught to reason like GPT-4, Meta’s LLaMA models which come in various sizes and have outperformed much larger offerings by OpenAI and Google, or Hugging Face’s BLOOM model, which is now available via Amazon’s Cloud Service AWS, now there are several powerful and free models that developers can build upon.
By providing such a robust foundation for free, these models have made immense cost reductions possible, while also aiding the proliferation of a wide gamut of AI tools and software. Due to all the above trends, innovation in AI has no longer remained the preserve of only the largest tech firms.
To be or not to be
We can see that there has been a fork in the road when it comes to AI development. While, on the one hand, large firms have continued to chase ever larger data and parameter sizes to improve their models, on the other hand, many factors are also helping develop increasingly competent yet smaller AI systems.
Which direction we tend towards in the future will depend on the nature of limitations that we encounter. If the larger models hit performance, data, or cost constraints that they cannot overcome, the push for larger sizes will get subdued.
And if continuing optimisations cannot improve small AI performance beyond a threshold, then veering towards a more capital-intensive direction could be the trend in AI’s growth. For now, we stand in a state of imperfect balance, where no outcome is foregone.
Written by Srimant Mishra. Srimant is a computer science engineer from VIT university, Vellore, with a deep interest in the field of Artificial Intelligence. He is currently pursuing a law degree at Utkal University, Bhubaneshwar.
Views are personal and do not represent the stand of this publication.